session 5 part 1 cour
DownloadTélécharger
Actions
Vote :
ScreenshotAperçu
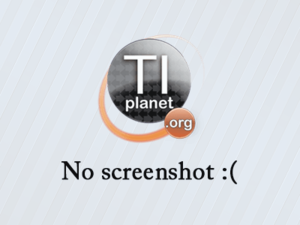
Informations
Catégorie :Category: nCreator TI-Nspire
Auteur Author: NSRBIKE
Type : Classeur 3.0.1
Page(s) : 1
Taille Size: 5.52 Ko KB
Mis en ligne Uploaded: 24/10/2024 - 06:58:21
Uploadeur Uploader: NSRBIKE (Profil)
Téléchargements Downloads: 1
Visibilité Visibility: Archive publique
Shortlink : http://ti-pla.net/a4272507
Type : Classeur 3.0.1
Page(s) : 1
Taille Size: 5.52 Ko KB
Mis en ligne Uploaded: 24/10/2024 - 06:58:21
Uploadeur Uploader: NSRBIKE (Profil)
Téléchargements Downloads: 1
Visibilité Visibility: Archive publique
Shortlink : http://ti-pla.net/a4272507
Description
Fichier Nspire généré sur TI-Planet.org.
Compatible OS 3.0 et ultérieurs.
<<
SAMPLING IS THE BASIS OF STATISTICAL INFERENCE " Sampling consists on extracting or measuring a random subset of data coming from a larger population. " Using this subsample of data, we would like to estimates features or statistics about the whole data. " It is a form of prediction , (but instead of predicting the relationship between two variables, we are predicting some intrinsic properties of one single variable, for instance its average value). " All our conclussions need to be given with a certain level of confidence. The larger the sample, the higher our confidence in the inference. DATABASES VS PHYSICAL DATA COLLECTION If we already have a lot of data, the process of sampling may consist simply on extracting a subset of our database (example: recommendation system in amazon) However, for some studies we need to collect the data physically. For instance: - Asking people on the street to test a new coffee brand - Physically testing a manufactured piece The way we collect this data is going to affect the quality and generality of my model Creating a suitable subsample for inference is more challenging than it may initially seem Different sampling methodologies offer various benefits but also come with potential biases.Below are some common sampling methods, their advantages, and associated risks: ### 1. **Random Sampling** Random sampling involves selecting a sample entirely at random. This method is easy to implement and cost-efficient, providing an objective way to select data. However, it may unintentionally overlook cases with low representation, leading to underrepresentation of certain groups. For example, a random database query based on randomly selected IDs may ignore smaller subgroups in the population. ### 2. **Systematic Sampling** In systematic sampling, data is selected at regular intervals. This method is simple and often produces a representative sample. However, if there are hidden cycles or patterns in the data, the sample might not fully represent the population. For instance, selecting sales data every Monday over the past four years might not be representative if Mondays are atypical for sales patterns. ### 3. **Quota Sampling** Quota sampling is a deterministic approach where the subsample is designed to maintain the same proportions of key characteristics as the overall population. This ensures that all subgroups are adequately represented. The downside is that subjectivity may arise when deciding which groups should be included. For example, when studying consumer behavior, identifying the profession of buyers and ensuring the sample reflects the population's professional distribution can introduce bias in defining the relevant categories. Each of these sampling methods has its own strengths and weaknesses, and the choice of method depends on the specific context and goals of the study. SAMPLING DISTRIBUTIONS. USEFUL FACTS Consider a univariate population with mean ¼ and standard deviation Ã. Lets take samples of size n. Then: ¡ If a population is normally distributed, the sampling distribution of the mean is also normally distributed ¡ With large samples (more than 30) the sampling distribution of the mean is approximately normally distributed regardless of the population distribution. ¡ In the two previous cases, the sampling distribution of the sample means has a mean ¼ and standard deviation Smaller interval => less chance to be there => less confidence (but more precission) ® Biggest interval => more chance to be there => more confidence (but less precission) Important fact to remember. The distribution of samples means (when N is large) is approximately a normal distribution. from scipy import stats ci_75 = stats.norm.interval(0.75) ci_80 = stats.norm.interval(0.8) ci_90 = stats.norm.interval(0.9) ci_95 = stats.norm.interval(0.95) print('75% interval: ', ci_75) print('80% interval: ', ci_80) print('90% interval: ', ci_90) print('95% interval: ', ci_95) 75% interval: (-1.1503493803760079, 1.1503493803760079) 80% interval: (-1.2815515655446004, 1.2815515655446004) 90% interval: (-1.6448536269514729, 1.6448536269514722) 95% interval: (-1.959963984540054, 1.959963984540054) Confidence Intervals for non standard normal distributions Suppose that I am sampling data from a distribution with mean=30 and standard deviation=5, and the size of each sample is N=50 What is a 95% confidence interval for the sample mean? Step 1: Find the value for the 95% ci of the standard normal distribution => 1.96 Step 2: Compute the standard error 5`5`5`5` = 55 5A5A = 5/50 = 0.7 Step 3: Create an interval centered around the mean using the previous values 56565656 = 30 1.96 × 0.7 , 30 + 1.96 × 0.7 = [ 5Ð5Ð5Ð5Ð. 5Ô5Ô5Ð5Ð5Ð5Ð , 5Ñ5Ñ5Ñ5Ñ. 5Ñ5Ñ5Ñ5Ñ5Ð5Ð ] Voici le texte en version simple pour que vous puissiez le copier : Dans l'exemple de Netflix, l'intervalle de confiance à 95% pour la moyenne de l'échantillon est calculé comme suit : 1. **Moyenne de la population (5)** : La moyenne est de
[...]
>>
Compatible OS 3.0 et ultérieurs.
<<
SAMPLING IS THE BASIS OF STATISTICAL INFERENCE " Sampling consists on extracting or measuring a random subset of data coming from a larger population. " Using this subsample of data, we would like to estimates features or statistics about the whole data. " It is a form of prediction , (but instead of predicting the relationship between two variables, we are predicting some intrinsic properties of one single variable, for instance its average value). " All our conclussions need to be given with a certain level of confidence. The larger the sample, the higher our confidence in the inference. DATABASES VS PHYSICAL DATA COLLECTION If we already have a lot of data, the process of sampling may consist simply on extracting a subset of our database (example: recommendation system in amazon) However, for some studies we need to collect the data physically. For instance: - Asking people on the street to test a new coffee brand - Physically testing a manufactured piece The way we collect this data is going to affect the quality and generality of my model Creating a suitable subsample for inference is more challenging than it may initially seem Different sampling methodologies offer various benefits but also come with potential biases.Below are some common sampling methods, their advantages, and associated risks: ### 1. **Random Sampling** Random sampling involves selecting a sample entirely at random. This method is easy to implement and cost-efficient, providing an objective way to select data. However, it may unintentionally overlook cases with low representation, leading to underrepresentation of certain groups. For example, a random database query based on randomly selected IDs may ignore smaller subgroups in the population. ### 2. **Systematic Sampling** In systematic sampling, data is selected at regular intervals. This method is simple and often produces a representative sample. However, if there are hidden cycles or patterns in the data, the sample might not fully represent the population. For instance, selecting sales data every Monday over the past four years might not be representative if Mondays are atypical for sales patterns. ### 3. **Quota Sampling** Quota sampling is a deterministic approach where the subsample is designed to maintain the same proportions of key characteristics as the overall population. This ensures that all subgroups are adequately represented. The downside is that subjectivity may arise when deciding which groups should be included. For example, when studying consumer behavior, identifying the profession of buyers and ensuring the sample reflects the population's professional distribution can introduce bias in defining the relevant categories. Each of these sampling methods has its own strengths and weaknesses, and the choice of method depends on the specific context and goals of the study. SAMPLING DISTRIBUTIONS. USEFUL FACTS Consider a univariate population with mean ¼ and standard deviation Ã. Lets take samples of size n. Then: ¡ If a population is normally distributed, the sampling distribution of the mean is also normally distributed ¡ With large samples (more than 30) the sampling distribution of the mean is approximately normally distributed regardless of the population distribution. ¡ In the two previous cases, the sampling distribution of the sample means has a mean ¼ and standard deviation Smaller interval => less chance to be there => less confidence (but more precission) ® Biggest interval => more chance to be there => more confidence (but less precission) Important fact to remember. The distribution of samples means (when N is large) is approximately a normal distribution. from scipy import stats ci_75 = stats.norm.interval(0.75) ci_80 = stats.norm.interval(0.8) ci_90 = stats.norm.interval(0.9) ci_95 = stats.norm.interval(0.95) print('75% interval: ', ci_75) print('80% interval: ', ci_80) print('90% interval: ', ci_90) print('95% interval: ', ci_95) 75% interval: (-1.1503493803760079, 1.1503493803760079) 80% interval: (-1.2815515655446004, 1.2815515655446004) 90% interval: (-1.6448536269514729, 1.6448536269514722) 95% interval: (-1.959963984540054, 1.959963984540054) Confidence Intervals for non standard normal distributions Suppose that I am sampling data from a distribution with mean=30 and standard deviation=5, and the size of each sample is N=50 What is a 95% confidence interval for the sample mean? Step 1: Find the value for the 95% ci of the standard normal distribution => 1.96 Step 2: Compute the standard error 5`5`5`5` = 55 5A5A = 5/50 = 0.7 Step 3: Create an interval centered around the mean using the previous values 56565656 = 30 1.96 × 0.7 , 30 + 1.96 × 0.7 = [ 5Ð5Ð5Ð5Ð. 5Ô5Ô5Ð5Ð5Ð5Ð , 5Ñ5Ñ5Ñ5Ñ. 5Ñ5Ñ5Ñ5Ñ5Ð5Ð ] Voici le texte en version simple pour que vous puissiez le copier : Dans l'exemple de Netflix, l'intervalle de confiance à 95% pour la moyenne de l'échantillon est calculé comme suit : 1. **Moyenne de la population (5)** : La moyenne est de
[...]
>>