FAMA FRENCH + MEAN VARIANCE IMPLEMATION THEORY
DownloadTélécharger
Actions
Vote :
ScreenshotAperçu
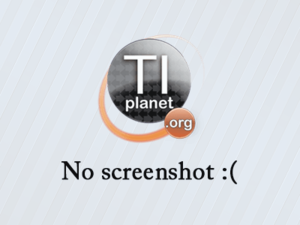
Informations
Catégorie :Category: nCreator TI-Nspire
Auteur Author: SPITZER2001
Type : Classeur 3.0.1
Page(s) : 1
Taille Size: 5.61 Ko KB
Mis en ligne Uploaded: 21/11/2024 - 21:04:04
Uploadeur Uploader: SPITZER2001 (Profil)
Téléchargements Downloads: 2
Visibilité Visibility: Archive publique
Shortlink : http://ti-pla.net/a4333616
Type : Classeur 3.0.1
Page(s) : 1
Taille Size: 5.61 Ko KB
Mis en ligne Uploaded: 21/11/2024 - 21:04:04
Uploadeur Uploader: SPITZER2001 (Profil)
Téléchargements Downloads: 2
Visibilité Visibility: Archive publique
Shortlink : http://ti-pla.net/a4333616
Description
Fichier Nspire généré sur TI-Planet.org.
Compatible OS 3.0 et ultérieurs.
<<
FAMA FRENCH + MEAN VARIANCE IMPLEMATION THEORY Long-short value-growth strategy : = Hi 10 - Lo 10 Multifactor portfolio : commentaire du prof sur le doc excel Long-short : From the chapter on mean-variance optimization with a risk-free asset, the excess return over the risk-free rate of a portfolio invested in risky and risk-free assets is the weighted average of the constituents' excess returns: 5_ _5]=5^2 5 The equally weighted portfolio is rebalanced every month, so it is buy-and-hold within one month, and its monthly excess return is the equally weighted average of the excess returns on the 3 constituents. This allows us to fill in column E. The above chart shows that the factor with the highest Sharpe ratio depends on the sample. In recent years, it is the market that had the best risk-adjusted return. The equally weighted portfolio of the 3 factors does not always dominate the 3 constituents, but it has always dominated the worst factor. Therefore, constructing a simple diversified portfolio of factors avoids taking a bet on which factor will deliver the best Sharpe ratio, and therefore avoids investing in the wrong factor.In particular, while every factor had at least one period of negative excess returns, the multifactor portfolio had always positive excess returns, thanks to a smoothing effect between the high and low returns of different factors. Long-only : Long-only portfolios are more correlated with one another than long-short portfolios because they all contain a significant exposure to the market factor. Therefore, the Sharpe ratios of the long-only factors are less dispersed than those of the long-short ones. But the Sharpe ratio of the multifactor portfolio was always above the the Sharpe ratio of the worst factor, meaning that the multifactor allocation avoids the risk of betting on the wrong factor. Moreover, like the portfolio of long-short size and value factors, the portfolio of long-only factors has always delivered a positive excess return in this sample. Fama-French data : EW : = MOYENNE(colonnes Mkt-Rf, SMB, HML) Fama-French regressions (multivariate regression) : =DROITEREG(colonne de excess return ; (colonne de MKT Rf ,SMB, HML groupé dun coup ) ; ;VRAI) Ex-post factor premia : Value : =MOYENNE( COLONNE HML) Size : =MOYENNE( COLONNE SMB) Market : =MOYENNE( COLONNE Mkt-RF) Return decomposition : Sample average : =MOYENNE( colonne de excess return) FF3-implied excess return : = SOMMEPROD(Valeurs estimates dans fama French regression des facteurs Value Size Market * Ex- post factor premier(Value, size, market) Alpha : Alpha estimate dans fama french regression FF3-implied + Alpha : Somme des deux precedentes SAMPLE AVERAGE RETURNS : Monthly : Average : MOYENNE (Monthlyreturn) Volatility : =ECARTYPE.PEARSON(Monthlyreturn) 95% lower bound monthy : = Average -1,96* Volatility /RACINE(NB(Monthlyreturn)) 95% upper bound monthy : = Average + 1,96* Volatility /RACINE(NB(Monthlyreturn)) Annualized : Average : = (1+ (Average : MOYENNE (Monthlyreturn))^12-1 95% lower bound anuallized : = (1+ 95% lower bound monthy )^12-1 95% upper bound anuallized : = (1+ 95% upper bound monthy )^12-1 Covariance Matrix : Regressions : Factor variance : =VAR.P.N((Mkt-factor)/100) Beta : = PENTE(Simple returns; ((Mkt-factor)/100) R-squared : = COEFFICIENT.DETERMINATION( Simple returns; ((Mkt-factor)/100)) Total variance : = VAR.P.N( Simple returns) Idiosyncratic variance : =(1- R-squared )* Total variance Systematic covariance matrix : ='Single index'! Factor variance *PRODUITMAT(TRANSPOSE(All betas );All betas ) Idiosyncratic covariance matrix : = Valeur de Idiosyncratic variance de chaque (action ou chaque portfeuille) dans les diagonales Covariance matrice estimates : = Systematic covariance matrix + covariance matrix estimates Constant correlation : Average correlation : =SOMME((correlation matric with data analysis tool)*(Mask( cellule ou il ya des valeurs dans la correlation matrix remplacer par des 1 ))/SOMME((Mask( cellule ou il ya des valeurs dans la correlation matrix remplacer par des 1 )) Constant correlation matrix : 1 dans les diagonales et valeur average correlation dans les autres valeurs Sample volatilities : =ECARTYPE.PEARSON(Simple returns) Diagonal matrix of volatilities : Valeurs de sample volatilities dans les diagonales Covariance matrix estimate : =PRODUITMAT(Diagonal matrix of volatilities;PRODUITMAT(Constant correlation matrix ; Diagonal matrix of volatilities)) Dans l'activité que le prof a fait il a construit portfolio gmv avec les differents type de covariance matrices (structured) et avec la sample( la classique quoi) le commentaire suivant explique les differences dans les gmv portfolio construit chacun grace chacune The differences between the results based on the use of the sample covariance matrix versus the single index and constant correlation estimators highlight the trade-offs between parsimony and robustne
[...]
>>
Compatible OS 3.0 et ultérieurs.
<<
FAMA FRENCH + MEAN VARIANCE IMPLEMATION THEORY Long-short value-growth strategy : = Hi 10 - Lo 10 Multifactor portfolio : commentaire du prof sur le doc excel Long-short : From the chapter on mean-variance optimization with a risk-free asset, the excess return over the risk-free rate of a portfolio invested in risky and risk-free assets is the weighted average of the constituents' excess returns: 5_ _5]=5^2 5 The equally weighted portfolio is rebalanced every month, so it is buy-and-hold within one month, and its monthly excess return is the equally weighted average of the excess returns on the 3 constituents. This allows us to fill in column E. The above chart shows that the factor with the highest Sharpe ratio depends on the sample. In recent years, it is the market that had the best risk-adjusted return. The equally weighted portfolio of the 3 factors does not always dominate the 3 constituents, but it has always dominated the worst factor. Therefore, constructing a simple diversified portfolio of factors avoids taking a bet on which factor will deliver the best Sharpe ratio, and therefore avoids investing in the wrong factor.In particular, while every factor had at least one period of negative excess returns, the multifactor portfolio had always positive excess returns, thanks to a smoothing effect between the high and low returns of different factors. Long-only : Long-only portfolios are more correlated with one another than long-short portfolios because they all contain a significant exposure to the market factor. Therefore, the Sharpe ratios of the long-only factors are less dispersed than those of the long-short ones. But the Sharpe ratio of the multifactor portfolio was always above the the Sharpe ratio of the worst factor, meaning that the multifactor allocation avoids the risk of betting on the wrong factor. Moreover, like the portfolio of long-short size and value factors, the portfolio of long-only factors has always delivered a positive excess return in this sample. Fama-French data : EW : = MOYENNE(colonnes Mkt-Rf, SMB, HML) Fama-French regressions (multivariate regression) : =DROITEREG(colonne de excess return ; (colonne de MKT Rf ,SMB, HML groupé dun coup ) ; ;VRAI) Ex-post factor premia : Value : =MOYENNE( COLONNE HML) Size : =MOYENNE( COLONNE SMB) Market : =MOYENNE( COLONNE Mkt-RF) Return decomposition : Sample average : =MOYENNE( colonne de excess return) FF3-implied excess return : = SOMMEPROD(Valeurs estimates dans fama French regression des facteurs Value Size Market * Ex- post factor premier(Value, size, market) Alpha : Alpha estimate dans fama french regression FF3-implied + Alpha : Somme des deux precedentes SAMPLE AVERAGE RETURNS : Monthly : Average : MOYENNE (Monthlyreturn) Volatility : =ECARTYPE.PEARSON(Monthlyreturn) 95% lower bound monthy : = Average -1,96* Volatility /RACINE(NB(Monthlyreturn)) 95% upper bound monthy : = Average + 1,96* Volatility /RACINE(NB(Monthlyreturn)) Annualized : Average : = (1+ (Average : MOYENNE (Monthlyreturn))^12-1 95% lower bound anuallized : = (1+ 95% lower bound monthy )^12-1 95% upper bound anuallized : = (1+ 95% upper bound monthy )^12-1 Covariance Matrix : Regressions : Factor variance : =VAR.P.N((Mkt-factor)/100) Beta : = PENTE(Simple returns; ((Mkt-factor)/100) R-squared : = COEFFICIENT.DETERMINATION( Simple returns; ((Mkt-factor)/100)) Total variance : = VAR.P.N( Simple returns) Idiosyncratic variance : =(1- R-squared )* Total variance Systematic covariance matrix : ='Single index'! Factor variance *PRODUITMAT(TRANSPOSE(All betas );All betas ) Idiosyncratic covariance matrix : = Valeur de Idiosyncratic variance de chaque (action ou chaque portfeuille) dans les diagonales Covariance matrice estimates : = Systematic covariance matrix + covariance matrix estimates Constant correlation : Average correlation : =SOMME((correlation matric with data analysis tool)*(Mask( cellule ou il ya des valeurs dans la correlation matrix remplacer par des 1 ))/SOMME((Mask( cellule ou il ya des valeurs dans la correlation matrix remplacer par des 1 )) Constant correlation matrix : 1 dans les diagonales et valeur average correlation dans les autres valeurs Sample volatilities : =ECARTYPE.PEARSON(Simple returns) Diagonal matrix of volatilities : Valeurs de sample volatilities dans les diagonales Covariance matrix estimate : =PRODUITMAT(Diagonal matrix of volatilities;PRODUITMAT(Constant correlation matrix ; Diagonal matrix of volatilities)) Dans l'activité que le prof a fait il a construit portfolio gmv avec les differents type de covariance matrices (structured) et avec la sample( la classique quoi) le commentaire suivant explique les differences dans les gmv portfolio construit chacun grace chacune The differences between the results based on the use of the sample covariance matrix versus the single index and constant correlation estimators highlight the trade-offs between parsimony and robustne
[...]
>>