ML
DownloadTélécharger
Actions
Vote :
ScreenshotAperçu
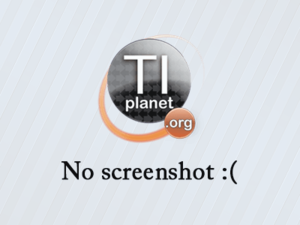
Informations
Catégorie :Category: nCreator TI-Nspire
Auteur Author: gadder
Type : Classeur 3.0.1
Page(s) : 1
Taille Size: 4.54 Ko KB
Mis en ligne Uploaded: 19/12/2024 - 15:36:55
Uploadeur Uploader: gadder (Profil)
Téléchargements Downloads: 3
Visibilité Visibility: Archive publique
Shortlink : http://ti-pla.net/a4424335
Type : Classeur 3.0.1
Page(s) : 1
Taille Size: 4.54 Ko KB
Mis en ligne Uploaded: 19/12/2024 - 15:36:55
Uploadeur Uploader: gadder (Profil)
Téléchargements Downloads: 3
Visibilité Visibility: Archive publique
Shortlink : http://ti-pla.net/a4424335
Description
Fichier Nspire généré sur TI-Planet.org.
Compatible OS 3.0 et ultérieurs.
<<
Exam Practice Statements with Correct Answers Highlighted 1. Lasso and Ridge Regularization Ï If the shrinkage parameter increases, the Lasso will select less / more features. (Correct Answer: less) 2. Bias-Variance Tradeoff Ï If you have overfitting, then you have less / more bias . (Correct Answer: less bias) 3. Data Leakage Ï Data leakage occurs when the test / training set is used to estimate hyperparameters. (Correct Answer: test) 4. Bayesian Classification Ï In Bayesian classification, we allocate an instance to the label that has the largest prior / posterior probability. (Correct Answer: posterior) 5. R-Squared Value Ï The test R-squared value may be negative: YES / NO. (Correct Answer: YES) 6. Learning Rate and Overfitting Ï If the learning rate increases, then the risk of overfitting decreases / increases . (Correct Answer: increases) 7. Random Forest Ï In Random Forest, we take bootstrap samples of inputs / instances . (Correct Answer: instances) 8. Adaboost Ï The Adaboost method gives more weight to correctly / misclassified instances. (Correct Answer: misclassified) 9. Naïve Bayes Ï Naïve Bayes is called "Naïve" because it assumes independence between features: YES / NO. (Correct Answer: YES) 10. Loss Function Ï If you use the exponential loss function, the loss of a correctly classified observation is strictly positive / zero . (Correct Answer: zero)11. Bootstrap Samples Ï When we have a sample of size 100, we expect the number of elements not in the bootstrap sample to be approximately 10 / 37 . (Correct Answer: 37) 12. Mean Absolute Error (MAE) Ï We would like the Mean Absolute Error (MAE) to be large / small . (Correct Answer: small) 13. Leave-One-Out Cross-Validation Ï For a training set of 50 instances, leave-one-out cross-validation requires training the model 49 / 50 times. (Correct Answer: 50) 14. Precision and Recall Ï Out of 100 persons with a disease, only 80 are predicted to have it. The recall / precision is 80%. (Correct Answer: Recall) 15. Regression Trees Ï Regression trees predict by computing an average / majority vote over the instances in the leaf node. (Correct Answer: average) 16. Standardization Ï Standardization of features is only useful if the model is not invariant under scaling: YES / NO. (Correct Answer: YES) 17. Cross-Validation Ï In k-fold cross-validation, refers to the number of folds / instances. (Correct Answer: folds) 18. Feature Selection Ï Principal Component Analysis (PCA) is used for feature generation / dimensionality reduction . (Correct Answer: dimensionality reduction) 19. Gini Impurity Ï Gini Impurity measures the likelihood of a random misclassification: YES / NO. (Correct Answer: YES) 20. Gradient Boosting Ï Gradient Boosting minimizes errors by focusing on residuals / probabilities. (Correct Answer: residuals) 21. Logistic Regression Ï Logistic regression outputs probabilities / distances. (Correct Answer: probabilities) 22. Overfitting Prevention Ï Adding more training data prevents overfitting: YES / NO. (Correct Answer: YES) 23. Decision Trees Ï Pruning a decision tree helps reduce overfitting: YES / NO. (Correct Answer: YES) 24. Ensemble Models Ï Bagging reduces variance / bias. (Correct Answer: variance) 25. Predictive Models Ï A predictive model with high recall minimizes false positives / false negatives . (Correct Answer: false negatives) 26. Hyperparameter Tuning Ï Grid search is used for model evaluation / hyperparameter tuning . (Correct Answer: hyperparameter tuning) 27. Regularization Ï Ridge regression penalizes large weights / small weights. (Correct Answer: large weights) 28. Neural Networks Ï ReLU is a linear / non-linear activation function. (Correct Answer: non-linear) 29. Data Imputation Ï Missing values can be handled using interpolation / imputation . (Correct Answer: imputation) 30. Outliers Ï The Mahalanobis distance is used to detect outliers in high-dimensional data: YES / NO. (Correct Answer: YES) 31. Correlation Ï Correlation captures linear / non-linear relationships. (Correct Answer: linear) 32. Clustering Ï K-means clustering requires the number of clusters to be specified: YES / NO. (Correct Answer: YES) 33. PCA Ï PCA projects data onto a higher / lower-dimensional space. (Correct Answer: lower-dimensional) 34. Variance Explained Ï The sum of explained variance by all principal components is equal to 1 / 100% . (Correct Answer: 100%) 35. Classification Metrics Ï F1 score is the harmonic mean of precision and recall: YES / NO. (Correct Answer: YES) 36. ROC Curve Ï The area under the ROC curve (AUC) ranges from 0 to 1 / 100 . (Correct Answer: 1) 37. K-Nearest Neighbors (KNN) Ï KNN requires labeled / unlabeled data for training. (Correct Answer: labeled) 38. Overfitting Detection Ï Cross-validation can help detect overfitting: YES / NO. (Correct Answer: YES) 39. Sampling Ï Stratified sampling ensures class proportions are maintained: YES
[...]
>>
Compatible OS 3.0 et ultérieurs.
<<
Exam Practice Statements with Correct Answers Highlighted 1. Lasso and Ridge Regularization Ï If the shrinkage parameter increases, the Lasso will select less / more features. (Correct Answer: less) 2. Bias-Variance Tradeoff Ï If you have overfitting, then you have less / more bias . (Correct Answer: less bias) 3. Data Leakage Ï Data leakage occurs when the test / training set is used to estimate hyperparameters. (Correct Answer: test) 4. Bayesian Classification Ï In Bayesian classification, we allocate an instance to the label that has the largest prior / posterior probability. (Correct Answer: posterior) 5. R-Squared Value Ï The test R-squared value may be negative: YES / NO. (Correct Answer: YES) 6. Learning Rate and Overfitting Ï If the learning rate increases, then the risk of overfitting decreases / increases . (Correct Answer: increases) 7. Random Forest Ï In Random Forest, we take bootstrap samples of inputs / instances . (Correct Answer: instances) 8. Adaboost Ï The Adaboost method gives more weight to correctly / misclassified instances. (Correct Answer: misclassified) 9. Naïve Bayes Ï Naïve Bayes is called "Naïve" because it assumes independence between features: YES / NO. (Correct Answer: YES) 10. Loss Function Ï If you use the exponential loss function, the loss of a correctly classified observation is strictly positive / zero . (Correct Answer: zero)11. Bootstrap Samples Ï When we have a sample of size 100, we expect the number of elements not in the bootstrap sample to be approximately 10 / 37 . (Correct Answer: 37) 12. Mean Absolute Error (MAE) Ï We would like the Mean Absolute Error (MAE) to be large / small . (Correct Answer: small) 13. Leave-One-Out Cross-Validation Ï For a training set of 50 instances, leave-one-out cross-validation requires training the model 49 / 50 times. (Correct Answer: 50) 14. Precision and Recall Ï Out of 100 persons with a disease, only 80 are predicted to have it. The recall / precision is 80%. (Correct Answer: Recall) 15. Regression Trees Ï Regression trees predict by computing an average / majority vote over the instances in the leaf node. (Correct Answer: average) 16. Standardization Ï Standardization of features is only useful if the model is not invariant under scaling: YES / NO. (Correct Answer: YES) 17. Cross-Validation Ï In k-fold cross-validation, refers to the number of folds / instances. (Correct Answer: folds) 18. Feature Selection Ï Principal Component Analysis (PCA) is used for feature generation / dimensionality reduction . (Correct Answer: dimensionality reduction) 19. Gini Impurity Ï Gini Impurity measures the likelihood of a random misclassification: YES / NO. (Correct Answer: YES) 20. Gradient Boosting Ï Gradient Boosting minimizes errors by focusing on residuals / probabilities. (Correct Answer: residuals) 21. Logistic Regression Ï Logistic regression outputs probabilities / distances. (Correct Answer: probabilities) 22. Overfitting Prevention Ï Adding more training data prevents overfitting: YES / NO. (Correct Answer: YES) 23. Decision Trees Ï Pruning a decision tree helps reduce overfitting: YES / NO. (Correct Answer: YES) 24. Ensemble Models Ï Bagging reduces variance / bias. (Correct Answer: variance) 25. Predictive Models Ï A predictive model with high recall minimizes false positives / false negatives . (Correct Answer: false negatives) 26. Hyperparameter Tuning Ï Grid search is used for model evaluation / hyperparameter tuning . (Correct Answer: hyperparameter tuning) 27. Regularization Ï Ridge regression penalizes large weights / small weights. (Correct Answer: large weights) 28. Neural Networks Ï ReLU is a linear / non-linear activation function. (Correct Answer: non-linear) 29. Data Imputation Ï Missing values can be handled using interpolation / imputation . (Correct Answer: imputation) 30. Outliers Ï The Mahalanobis distance is used to detect outliers in high-dimensional data: YES / NO. (Correct Answer: YES) 31. Correlation Ï Correlation captures linear / non-linear relationships. (Correct Answer: linear) 32. Clustering Ï K-means clustering requires the number of clusters to be specified: YES / NO. (Correct Answer: YES) 33. PCA Ï PCA projects data onto a higher / lower-dimensional space. (Correct Answer: lower-dimensional) 34. Variance Explained Ï The sum of explained variance by all principal components is equal to 1 / 100% . (Correct Answer: 100%) 35. Classification Metrics Ï F1 score is the harmonic mean of precision and recall: YES / NO. (Correct Answer: YES) 36. ROC Curve Ï The area under the ROC curve (AUC) ranges from 0 to 1 / 100 . (Correct Answer: 1) 37. K-Nearest Neighbors (KNN) Ï KNN requires labeled / unlabeled data for training. (Correct Answer: labeled) 38. Overfitting Detection Ï Cross-validation can help detect overfitting: YES / NO. (Correct Answer: YES) 39. Sampling Ï Stratified sampling ensures class proportions are maintained: YES
[...]
>>