Implementation mean-variance theory
DownloadTélécharger
Actions
Vote :
ScreenshotAperçu
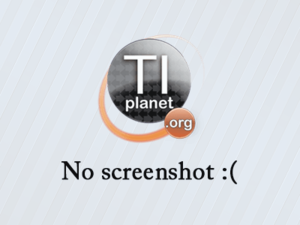
Informations
Catégorie :Category: nCreator TI-Nspire
Auteur Author: SPITZER2001
Type : Classeur 3.0.1
Page(s) : 1
Taille Size: 6.54 Ko KB
Mis en ligne Uploaded: 22/11/2024 - 06:20:42
Uploadeur Uploader: SPITZER2001 (Profil)
Téléchargements Downloads: 2
Visibilité Visibility: Archive publique
Shortlink : http://ti-pla.net/a4334864
Type : Classeur 3.0.1
Page(s) : 1
Taille Size: 6.54 Ko KB
Mis en ligne Uploaded: 22/11/2024 - 06:20:42
Uploadeur Uploader: SPITZER2001 (Profil)
Téléchargements Downloads: 2
Visibilité Visibility: Archive publique
Shortlink : http://ti-pla.net/a4334864
Description
Fichier Nspire généré sur TI-Planet.org.
Compatible OS 3.0 et ultérieurs.
<<
The Estimation Problem ¶ The tangency portfolio depends on the covariance matrix and the expected excess returns: 50tan = 5º15Í /5Ï25º15Í ¶ These parameters are not observed and must be estimated from past data, so we have an estimated tangency portfolio: 50tan = 5º1 5Í /5Ï2 5º1 5Í 5º = estimated covariance matrix; 5Í = estimated expected excess returns ¶ How good is this portfolio when we hold it out of the sample from which the parameters were estimated? Mean-Variance Optimization Versus Equal Weighting ¶ Reference paper: DeMiguel, Garlappi and Uppal (2009). Optimal Versus Naive Diversification: How Inefficient is the 1/5A Portfolio Strategy? Review of Financial Studies. ¶ The tangency portfolio is estimated with the sample covariance matrix and the sample average returns. ¶ The portfolio is rebalanced every month based on parameters estimated from the past 120 months of data. ¶ We compare it with the equally weighted portfolio, whose weights are 5d5V = 1/ 5A for every 5V = 1, 2, ..., 5A Mean-Variance Optimization Versus Equal Weighting ¶ Excerpt from Table 3 of DeMiguel et al. (2009): ¶ The equally weighted portfolio has higher Sharpe ratio than the mean-variance efficient portfolio in 5 out of 6 investment universes! ¶ mv (in sample) is the tangency portfolio estimated with the full sample of returns (unrealistic!) ¶ Equal weighting (naive diversification) performs often much better than mean-variance (scientific diversification)... How to Improve Mean-Variance Efficient Portfolios? ¶ Conclusion: Estimation errors penalize severely mean-variance efficient portfolios. ¶ Approach 1: Improve inputs (expected return and covariance estimates): ¶ Why do sample estimates perform poorly? ¶ Search for better parameter estimates. ¶ Approach 2: Regularize outputs (weights): ¶ Introduce weight constraints in mean-variance optimization to make efficient portfolios closer to equally weighted. ¶ Approach 3: Reduce the number of parameters to estimate: ¶ Use parsimonious diversification methods without expected returns, or without correlations, or without volatilities In this context, "parsimonious" refers to methods or approaches that aim to simplify the model by reducing the number of parameters to estimate. Parsimonious diversification methods focus on achieving effective diversification while avoiding the need to rely on complex inputs, such as expected returns, correlations, or volatilities, thus making the process more efficient and less prone to estimation errors. Sample Mean ¶ A natural estimate of the expected return 55V is the sample mean of past returns. Definition The sample mean of returns for asset 5V is 55V = 5_5V = 1 /5G 5G 5_5a,5V 5a=1 5_5a,5V ¶ How good is this as an estimate of the true expected return 55V The Sample Mean is Unbiased Property Assume that, for all date 5a, 5< [5_5a,5V] = 55V Then, 55V is an unbiased estimator of 55V. ¶ Interpretation: the average of sample means across multiple independent samples with the same size 5G converges to the true expected return as the number of samples grows to infinity (for fixed 5G). In essence, "unbiased" ensures that, on average, across many samples, the estimator provides a correct reflection of the true parameter. This property is critical for statistical inference, as it ensures reliability in the estimation process. Confidence Interval for the Expected Return Property Assume that the returns 5_0,5V, 5_1,5V, ..., 5_5a,5V, ... are independent and identically distributed with mean 55V and variance 52 5V . Then, an asymptotic 95% confidence interval for the expected return 55V estimated from 5G returns is [b5V, ub5V], where b5V = 55V 1.96 55V /5G , ub5V = 55V + 1.96 55V /5G and 55V is the sample volatility of returns. ¶ Interpretation: the true expected return lies within the confidence bounds with a probability that converges to 95% as the sample size 5G grows to infinity. Confidence bounds are very wide. ¶ Past average returns provide little information about expected returns. Estimating Expected Returns in Practice ¶ Approach 1: postulate some risk - expected return relationship. ¶ Scientific Beta Efficient Maximum Sharpe Ratio Indices assume that expected excess returns are proportional to semivolatilities; ¶ TOBAMs Maximum Diversification Approach assumes that expected excess returns are proportional to volatilities (see foundation paper by Choueifaty and Coignard (2008). Towards Maximum Diversification. Journal of Portfolio Management); ¶ Use a multifactor model, where expected returns are functions of betas (but this may still lead to unrealistic estimates). ¶ Approach 2 (beyond the scope of this course): incorporate prior views about expected returns. ¶ The Black-Litterman model (Black and Litterman (1992), Global Portfolio Optimization. Financial Analysts Journal) mixes investors views with expected returns inferred from the market portfolio. ¶ See workbook Black-Litterman.xlsx. ¶ Ap
[...]
>>
Compatible OS 3.0 et ultérieurs.
<<
The Estimation Problem ¶ The tangency portfolio depends on the covariance matrix and the expected excess returns: 50tan = 5º15Í /5Ï25º15Í ¶ These parameters are not observed and must be estimated from past data, so we have an estimated tangency portfolio: 50tan = 5º1 5Í /5Ï2 5º1 5Í 5º = estimated covariance matrix; 5Í = estimated expected excess returns ¶ How good is this portfolio when we hold it out of the sample from which the parameters were estimated? Mean-Variance Optimization Versus Equal Weighting ¶ Reference paper: DeMiguel, Garlappi and Uppal (2009). Optimal Versus Naive Diversification: How Inefficient is the 1/5A Portfolio Strategy? Review of Financial Studies. ¶ The tangency portfolio is estimated with the sample covariance matrix and the sample average returns. ¶ The portfolio is rebalanced every month based on parameters estimated from the past 120 months of data. ¶ We compare it with the equally weighted portfolio, whose weights are 5d5V = 1/ 5A for every 5V = 1, 2, ..., 5A Mean-Variance Optimization Versus Equal Weighting ¶ Excerpt from Table 3 of DeMiguel et al. (2009): ¶ The equally weighted portfolio has higher Sharpe ratio than the mean-variance efficient portfolio in 5 out of 6 investment universes! ¶ mv (in sample) is the tangency portfolio estimated with the full sample of returns (unrealistic!) ¶ Equal weighting (naive diversification) performs often much better than mean-variance (scientific diversification)... How to Improve Mean-Variance Efficient Portfolios? ¶ Conclusion: Estimation errors penalize severely mean-variance efficient portfolios. ¶ Approach 1: Improve inputs (expected return and covariance estimates): ¶ Why do sample estimates perform poorly? ¶ Search for better parameter estimates. ¶ Approach 2: Regularize outputs (weights): ¶ Introduce weight constraints in mean-variance optimization to make efficient portfolios closer to equally weighted. ¶ Approach 3: Reduce the number of parameters to estimate: ¶ Use parsimonious diversification methods without expected returns, or without correlations, or without volatilities In this context, "parsimonious" refers to methods or approaches that aim to simplify the model by reducing the number of parameters to estimate. Parsimonious diversification methods focus on achieving effective diversification while avoiding the need to rely on complex inputs, such as expected returns, correlations, or volatilities, thus making the process more efficient and less prone to estimation errors. Sample Mean ¶ A natural estimate of the expected return 55V is the sample mean of past returns. Definition The sample mean of returns for asset 5V is 55V = 5_5V = 1 /5G 5G 5_5a,5V 5a=1 5_5a,5V ¶ How good is this as an estimate of the true expected return 55V The Sample Mean is Unbiased Property Assume that, for all date 5a, 5< [5_5a,5V] = 55V Then, 55V is an unbiased estimator of 55V. ¶ Interpretation: the average of sample means across multiple independent samples with the same size 5G converges to the true expected return as the number of samples grows to infinity (for fixed 5G). In essence, "unbiased" ensures that, on average, across many samples, the estimator provides a correct reflection of the true parameter. This property is critical for statistical inference, as it ensures reliability in the estimation process. Confidence Interval for the Expected Return Property Assume that the returns 5_0,5V, 5_1,5V, ..., 5_5a,5V, ... are independent and identically distributed with mean 55V and variance 52 5V . Then, an asymptotic 95% confidence interval for the expected return 55V estimated from 5G returns is [b5V, ub5V], where b5V = 55V 1.96 55V /5G , ub5V = 55V + 1.96 55V /5G and 55V is the sample volatility of returns. ¶ Interpretation: the true expected return lies within the confidence bounds with a probability that converges to 95% as the sample size 5G grows to infinity. Confidence bounds are very wide. ¶ Past average returns provide little information about expected returns. Estimating Expected Returns in Practice ¶ Approach 1: postulate some risk - expected return relationship. ¶ Scientific Beta Efficient Maximum Sharpe Ratio Indices assume that expected excess returns are proportional to semivolatilities; ¶ TOBAMs Maximum Diversification Approach assumes that expected excess returns are proportional to volatilities (see foundation paper by Choueifaty and Coignard (2008). Towards Maximum Diversification. Journal of Portfolio Management); ¶ Use a multifactor model, where expected returns are functions of betas (but this may still lead to unrealistic estimates). ¶ Approach 2 (beyond the scope of this course): incorporate prior views about expected returns. ¶ The Black-Litterman model (Black and Litterman (1992), Global Portfolio Optimization. Financial Analysts Journal) mixes investors views with expected returns inferred from the market portfolio. ¶ See workbook Black-Litterman.xlsx. ¶ Ap
[...]
>>